David D. Breshears
Regents Professor Emeritus
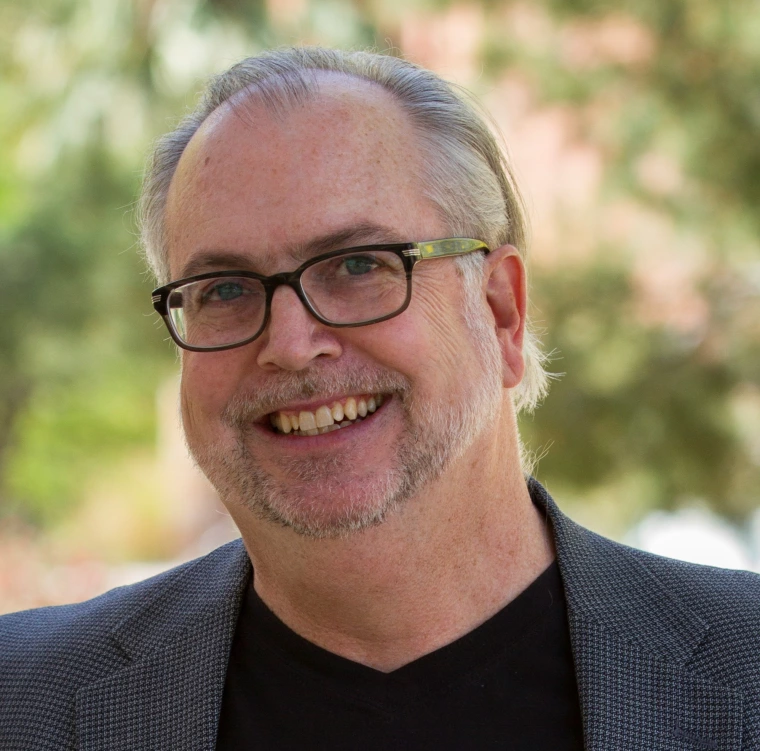
BSE 325H
1311 E 4th St
Tucson, AZ 85719
Research
Our research focuses on ecology-hydrology interrelationships, gradients from grassland to forest, transport and erosion of sediment by water and wind, and drought-induced tree die-off, all to aide in addressing climate change, land use, and pollution
Teaching
Classes taught include Dryland Ecohydrology & Vegetation Dynamics (WSM/RNR/EEB/HWRS 452/552), Leadership & Communication Skills for Environmental Scientists (RNR 596L), & National Climate Assessment (RNR 496G/596G) as well as frequent guest lectures.
Degree(s)
- BS (1985) Agriculture (Wildlife Science), New Mexico State University, Las Cruces, NM
- MS (1987) Radiology and Radiation Biology (Radioecology), Colorado State University, Fort Collins, CO
- PhD (1993) Radiological Health Sciences (Radioecology with Program in Ecological Studies), Colorado State University, Fort Collins, CO